๋ชฉ๋ก๐๐ช๐ฝ๐ฎ๐ฐ๐ธ๐ป๐ (3)
๐๐๐ ๐๐๐๐บ๐๐๐๐๐
In Python, "self" refers to the specific instance that is calling the method. So, "self" is used to assign or update attributes for the specific instance.# Defining a Class using "self"class greatatcoding: def __init__(self, name, age): self.name = name # "self.name" is an instance attribute; "name" is a local parameter self.age = age def hello(self): print(f"..
1. Using @torch.no_grad() It's a decorator used in Pytorch that disables automatic differentiation. This means that gradients are not calculated within the scope of this function.Since the goal is not to update model weights but rather generate results, it is primarily used during the inference phase.2. torch.randn() vs torch.randn_like() ~ to be continued ~ ~ gonna keep updating ~
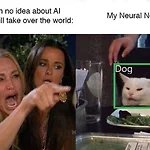
All seminar presentation materials will be uploaded to this blog, even if they happen to be private posts. The final script that would be uploaded together with the material should be fully written in English.Additionally, all feedbacks received from the seminar should be organized and summarized in English. AT LEAST.. once a month, I will be summarizing the materials that I'm studying at the mo..